Behnam Javanparast*, Mahmoud El Mabrouk, Estelle Inack
Introduction
Quantum Intelligence is a new and fast-growing field that has emerged from the advancements in Artificial Intelligence and Quantum Physics techniques (theoretical and experimental) in the past decade.
The quantum aspect of this field could be construed as either the use of quantum hardware, i.e. running algorithms on available quantum devices, the so-called Noisy-Intermediate Scale Quantum (NISQ) devices, or the use of Quantum-Inspired algorithms. These algorithms are inspired by Quantum Physics and have a track record of tackling complex computational physics problems on classical computers.
Although the efforts to build the first fault-tolerant universal quantum computer are at an all-time high, more progress is still needed to obtain commercial viability in surpassing the existing classical computational infrastructures. So the secret to taking advantage of the power of quantum today is Quantum-Inspired methods.
By combining artificial neural networks with Quantum-Inspired algorithms, we can build a more powerful tool, Quantum Intelligent algorithms, in which simulated quantum dynamics are boosted with the pattern recognition abilities of neural networks.
At yiyaniQ, we have been developing and testing proprietary Quantum Intelligent algorithms focusing on the Financial sector. In particular, we are currently interested in the problem of portfolio optimization. This problem reads as: given an amount of capital and a set of available assets, how to maximize return and minimize risk by allocating that capital amongst a certain subset of those assets. We have obtained results demonstrating the advantage of these classes of algorithms in the portfolio optimization problem. Our method is agnostic to how the assets’ time series are obtained, i.e. whether they are purely historical time series or generated using different predictive methods. This makes these algorithms compatible with other tools and techniques currently used within the financial sector.
Benchmarks
Stating the problem
We created a portfolio of S&P 100 stocks where we distribute the nominal capital amount of 1 dollar amongst these 100 stocks. Our inputs are the historical covariance matrix and returns, with a look-back window of 60 days. We also impose investment bands (minimum and maximum amount of investment) for each of the stocks in the portfolio. We then obtain the maximum Sharpe ratio portfolio for the next day.
We would like to emphasize that the input time series of the returns do not need to be historical. They could be forward-looking and generated with predictive methods of choice to which our algorithms are agnostic. We have used the following benchmarks:
Results
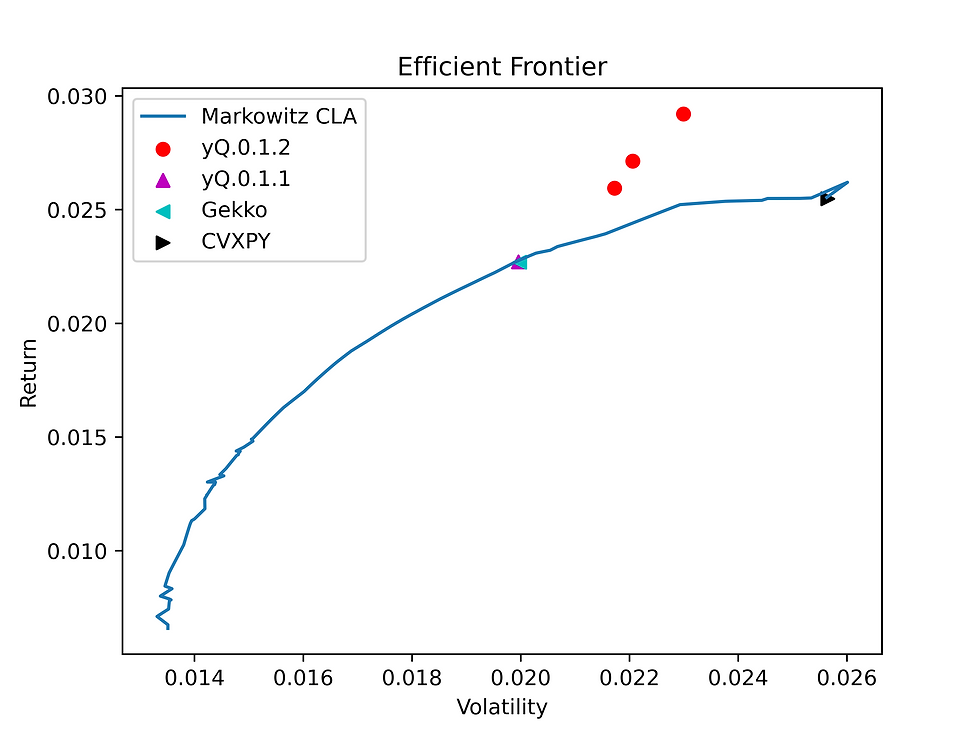
Fig.1 Red dots demonstrate portfolios constructed by quantum intelligent algorithms pushing the boundaries of efficient frontier.
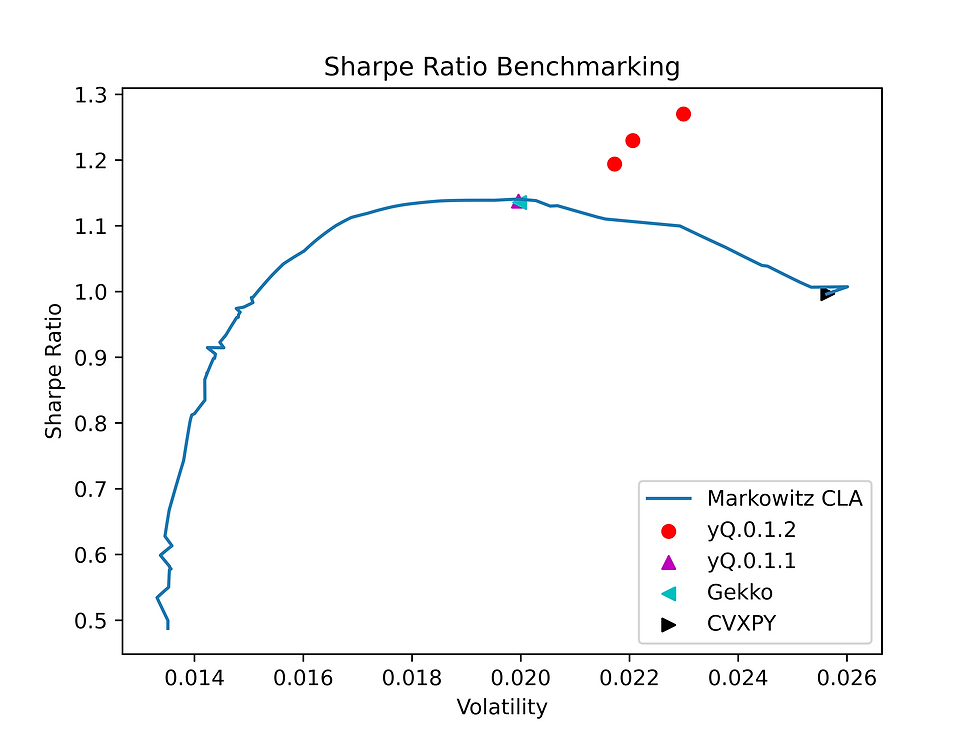
Fig2. Red dots represent quantum intelligent portfolio's’ Sharpe ratio. They surpassed the Sharpe ratios of all the benchmarks.
Table 1. Max Sharpe Ratio Portfolio Metrics. 1 to 3 tags for yQ.0.1.2 in this table represent the red dots in figures 1 and 2, from left to right. They are the best three solutions obtained with the yQ.0.1.2 optimizer.
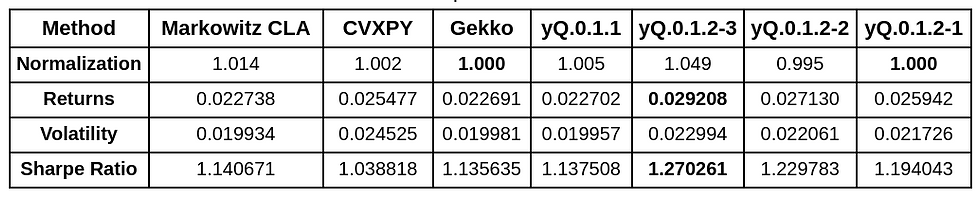
Analysis
yiyaniQ's Quantum Intelligent algorithms have shown better performance with respect to the benchmarks.
yQ algorithms achieved higher Sharpe ratios compared to both open source benchmarks while keeping capital distribution equally efficient (see the Normalization row in Table.1). Regarding Markowitz's efficient frontier, Quantum Intelligent algorithms were able to push the boundaries. The highest Sharpe Ratios rose as high as 8% while keeping the capital distribution more efficient than Markowitz’s maximum Sharpe ratio portfolio.
Tables 2 and 3 summarize our Quantum Intelligent algorithms' improvement to the benchmark portfolios.
Table 2. Capital Efficiency Improvements by Quantum Intelligence. Capital efficiency is the absolute value of the result of subtracting one from the normalization of each method. The improvement is the simple difference between the efficiency of the benchmark and the yQ algorithm multiplied by 100.
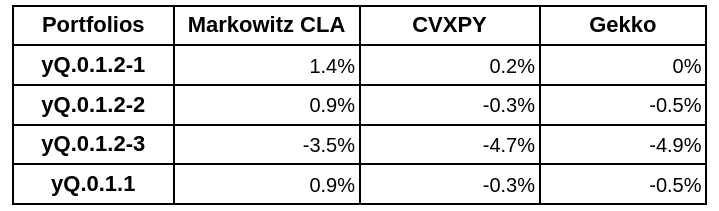
Table 3. Sharpe ratio Improvements by Quantum Intelligence.
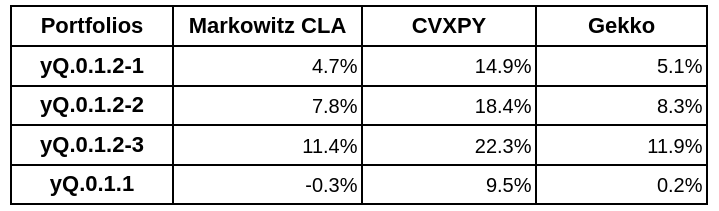
Our Quantum Intelligent algorithms are in their initial stages of development. The preliminary results presented here demonstrate the advantage they could bring to the portfolio optimization problem. We expect to see more improvements as we further explore existing algorithms and design new ones.
Quantum Intelligent Software
The above results show the advantage that Quantum Intelligent algorithms can bring to finance today. This is not limited to only improving the metrics presented above, although they are strong indicators of the potency of these methods. The flexibility and versatility of Quantum Intelligence in dealing with complexity is another important feature. They put more control in the hands of the users while being compatible with and complementary to the other tools they utilize. This in particular is manifested by the ease of implementing and investigating complex constraints related to the problem of interest. In our initial benchmarking, we first hand observed the shortcomings in considering for example non-linear constraints in some of the open-source softwares. Also, considering issues related to sparse matrix inversion in standard methods currently used for portfolio optimization, Quantum Intelligent algorithms offer a more powerful alternative that circumvents this issue.
The culmination of all these advantages is presented in a Quantum Intelligent software for portfolio optimization. That will give users the ability to encode constraints of their choice and obtain a plethora of near-optimal solutions that, in principle, could go through further selections depending on their preferences.
A note on the use of quantum hardware and quantum-readiness
yiyaniQ is focused on the Quantum-Inspired methods instead of using available quantum hardware to harvest the quantum advantage today. However, the Quantum Intelligent software could be designed to allow integration of the quantum hardware, making yiyaniQ quantum-ready. This goal is on the horizon for us when we receive strong signals that quantum hardware can deliver business value. At the time being, we believe Quantum-Inspired methods are the best alternative to quantum hardware, and they have shown strong capabilities presented above.
Comments